A pair of researchers at Northern Arizona University used GPU-based deep-learning algorithms to categorize sherds — tiny fragments of ancient pottery — as well as, or better than, four expert archaeologists.
The technique, outlined in a paper published in the June issue of The Journal of Archaeological Science by Leszek Pawlowicz and Christian Downum focused on a specific kind of ancient painted pottery from the American Southwest known as Tusayan White Ware.
This pottery ware, which features geometric black designs painted on white ceramics, was created in what is now northeastern Arizona between A.D. 825 and 1300. In the 1920s, archaeologists figured out how to use the designs to categorize the pottery so they can understand when artisans created each pottery fragment.
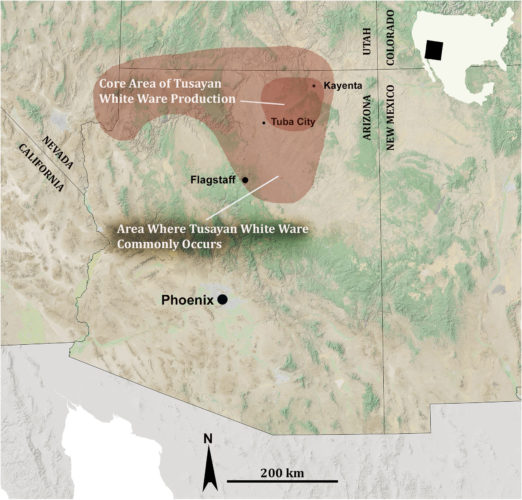
As a result, Tusayan White Ware sherds are a window into the sophisticated, preliterate cultures of the American Southwest. They represent just a sliver of the rich archaeological heritage of the southeastern United States.
“There are tens if not hundreds of thousands of sites that were occupied 1,500 years ago until modern times by the Hopi and the Zuni in Arizona in New Mexico,” explains Pawlowicz, an adjunct faculty member at Northern Arizona University (and a Jeopardy! Tournament of Champions winner in 1992).
The challenges archaeologists studying pottery sherds face parallels those confronted by scientists across a sprawling array of fields — from astronomy to zoology — who are turning to AI to harness larger and larger quantities of data to tackle increasingly ambitious projects.
Identifying Long-Dead Artisans
Downum, an anthropology professor at Northern Arizona University, says quickly analyzing sherds from the region — and worldwide — would allow researchers to glean new insights into life hundreds of years ago.
They might even be able to, one day, recognize the work of individual artisans and trace the distribution of pottery across long-dormant trading networks.
The problem is that very few have the training — and the decades of experience needed — to understand what they’re looking at.
For archaeologists, turnover is a concern. For example, there’s only one person still actively working who was trained by the first-generation definers of the design categories, and perhaps a dozen trained by the second generation of experts.
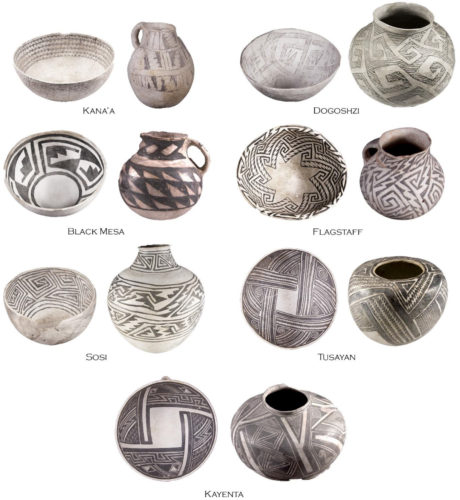
So digitizing hundreds of thousands of images of sherds, along with geographical information, would be a potent tool.
Human-Level Expertise
In their study, Pawlowicz and Downum showed that, when properly trained, a deep learning model can assign types to digital images of decorated sherds with an accuracy comparable to, and sometimes higher than, four expert-level contemporary archaeologists.
Pawlowicz trained the AI model used for the study in just a few hours on his PC, which is equipped with an NVIDIA GPU running a pair of common convolutional neural network models, VGG16 and ResNet50.
And the model, far from competing with existing archaeologists, could even be a valuable tool for training new ones.
More Explainable
Deep learning, Pawlowicz and Downum explain, has long been seen as a “black box.”
But new tools such as Grad-CAM (gradient-based class activation maps) “queries” the model to determine which areas of the input image were most important in assigning a type confidence to the image, Pawlowicz and Downum write.
The results are then displayed as a “heat map,” where areas most relevant to the type classification are shaded in red and less relevant areas in blue.
By contrast, human experts often struggle to point to the specific features in a sherd sample that led them to categorize, making it more challenging to explain their work and train others.
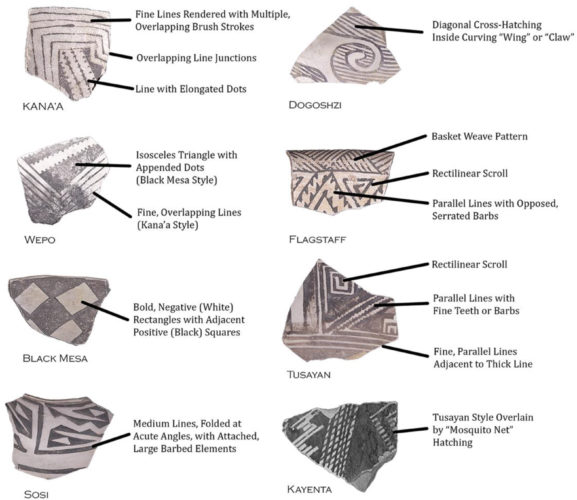
Consistency is another advantage. While one human expert will often classify a sherd differently from another human, an AI can measure consistently. Thus, even with a flawed yardstick, comparisons among vast quantities of samples with AI is possible and valuable.
Deep learning also makes it easier to apply new insights to sherd samples classified a century or more ago, using new concepts on older work.
Crowdsourced Archaeology?
Next, Pawlowicz plans to turn to NVIDIA-powered systems at Northern Arizona University to analyze even larger sample sets using more complex models.
Downum and Pawlowicz would like to create a central searchable database of images. Smartphone apps might even help people in the field with little experience add to the database — snapping photos of sherds in situ without disturbing them.
Once uploaded, AI would allow for expert analysis of every fragment. “There’s a huge potential for this,” Downum said.
Image credits: Leszek Pawlowicz and Christian Downum/Northern Arizona University