With coral reefs in rapid decline across the globe, researchers from the University of Hawaii at Mānoa have pioneered an AI-based surveying tool that monitors reef health from the sky.
Using deep learning models and high-resolution satellite imagery powered by NVIDIA GPUs, the researchers have developed a new method for spotting and tracking coral reef halos — distinctive rings of barren sand encircling reefs.
The study, recently published in the Remote Sensing of Environment journal, could unlock real-time coral reef monitoring and turn the tide on global conservation.
“Coral reef halos are a potential proxy for ecosystem health,” said Amelia Meier, a postdoctoral fellow at the University of Hawaii and co-author of the study. “Visible from space, these halo patterns give scientists and conservationists a unique opportunity to observe vast and distant areas. With AI, we can regularly assess halo presence and size in near real time to determine ecosystem well-being.”
Sea-ing Clearly: Illuminating Reef Health
Previously attributed solely to fish grazing, reef halos can also indicate a healthy predator-prey ecosystem, according to researchers’ recent discoveries. While some herbivorous fish graze algae or seagrass near the protective reef perimeter, hunters dig around the seafloor for burrowed invertebrates, laying bare the surrounding sand.
These dynamics indicate the area hosts a healthy food buffet for sustaining a diverse population of ocean dwellers. When the halo changes shape, it signals an imbalance in the marine food web and could indicate an unhealthy reef environment.
In Hot Water
While making up less than 1% of the ocean, coral reefs offer habitat, food and nursery grounds for over 1 million aquatic species. There’s also huge commercial value — about $375 billion annually in commercial and subsistence fishing, tourism and coastal storm protection, and providing antiviral compounds for drug discovery research.
However, reef health is threatened by overfishing, nutrient contamination and ocean acidification. Intensifying climate change — along with the resulting thermal stress from a warming ocean — also increases coral bleaching and infectious disease.
Over half of the world’s coral reefs are already lost or badly damaged, and scientists predict that by 2050 all reefs will face threats, with many in critical danger.
Charting New Horizons With AI
Spotting changes in reef halos is key to global conservation efforts. However, tracking these changes is labor- and time-intensive, limiting the number of surveys that researchers can perform every year. Access to reefs in remote locations also poses challenges.
The researchers created an AI tool that identifies and measures reef halos from global satellites, giving conservationists an opportunity to proactively address reef degradation.
Using Planet SkySat images, they developed a dual-model framework employing two types of convolutional neural networks (CNNs). Relying on computer vision methods for image segmentation, they trained a Mask R-CNN model that detects the edges of the reef and halo, pixel by pixel. A U-Net model trained to differentiate between the coral reef and halo then classifies and predicts the areas of both.
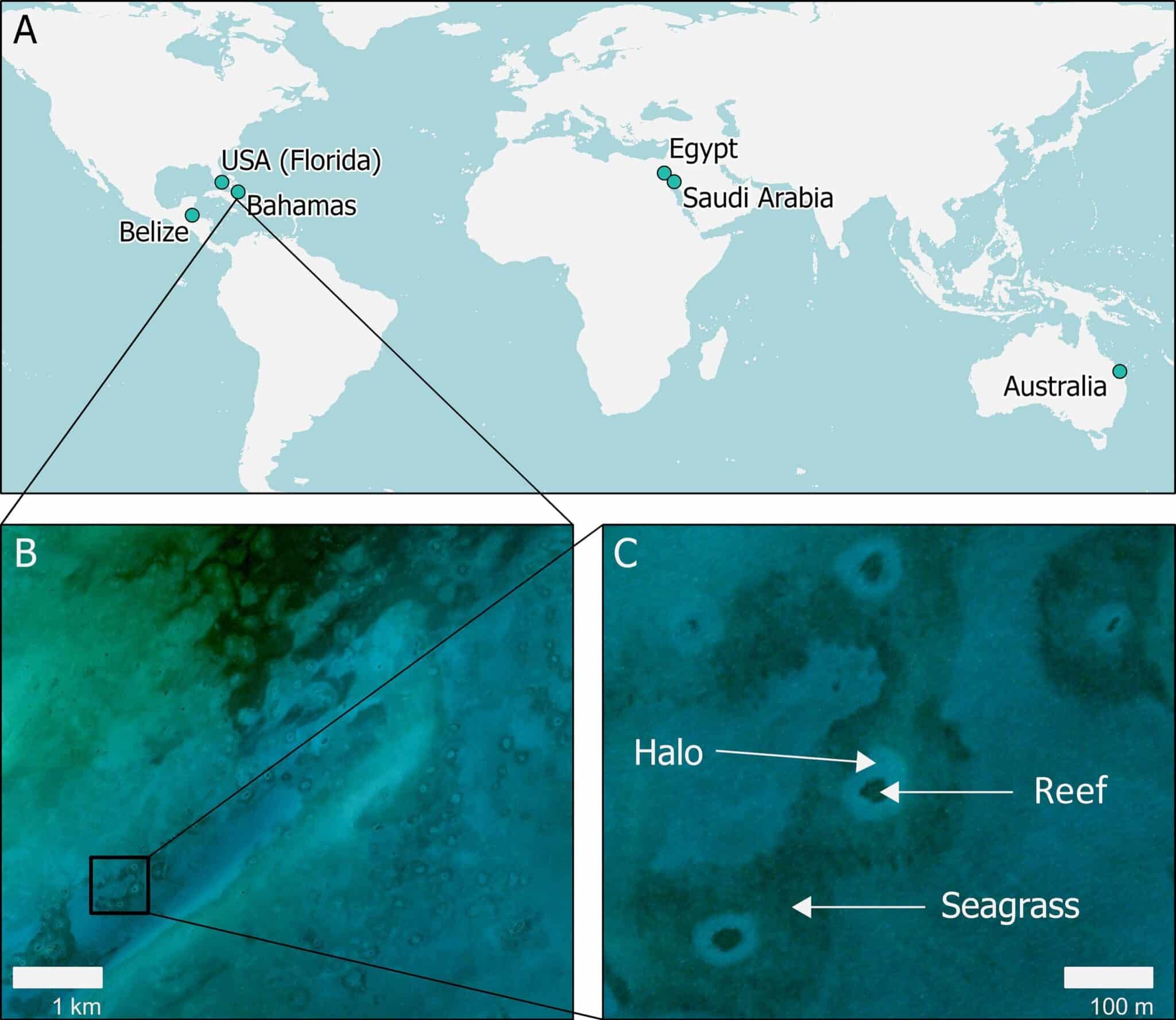
The team used TensorFlow, Keras and PyTorch libraries for training and testing thousands of annotations on the coral reef models.
To handle the task’s large compute requirements, the CNNs operate on an NVIDIA RTX A6000 GPU, boosted by a cuDNN-accelerated PyTorch framework. The researchers received the A6000 GPU as participants in the NVIDIA Academic Hardware Grant Program.
The AI tool quickly identifies and measures around 300 halos across 100 square kilometers in about two minutes. The same task takes a human annotator roughly 10 hours. The model also reaches about 90% accuracy depending on location and can navigate various and complicated halo patterns.
“Our study marks the first instance of training AI on reef halo patterns, as opposed to more common AI datasets of images, such as those of cats and dogs,” Meier said. “Processing thousands of images can take a lot of time, but using the NVIDIA GPU sped up the process significantly.”
One challenge is that image resolution can be a limiting factor in the model’s accuracy. Coarse-scale imagery with low resolutions makes it difficult to spot reef and halo boundaries and creates less accurate predictions.
Shoring Up Environmental Monitoring
“Our long-term goal is to transform our findings into a robust monitoring tool for assessing changes in halo size and to draw correlations to the population dynamics of predators and herbivores in the area,” Meier said.
With this new approach, the researchers are exploring the relationship between species composition, reef health, and halo presence and size. Currently, they’re looking into the association between sharks and halos. If their hypothesized predator-prey-halo interaction proves true, the team anticipates estimating shark abundance from space.