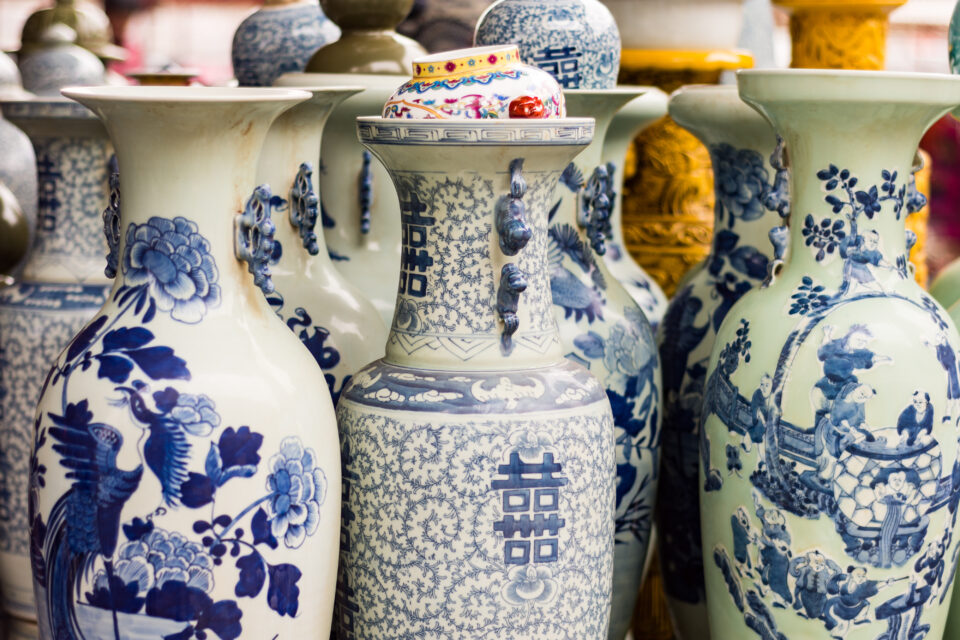
Ceramics — the humble mix of earth, fire and artistry — have been part of a global conversation… Read Article
Ceramics — the humble mix of earth, fire and artistry — have been part of a global conversation… Read Article
As one of the world’s largest emerging markets, Indonesia is making strides toward its “Golden 2045 Vision” — an initiative tapping digital technologies and bringing together government, enterprises, startups and… Read Article
In many parts of the world, including major technology hubs in the U.S., there’s a yearslong wait for AI factories to come online, pending the buildout of new energy infrastructure… Read Article
To speed up AI adoption across industries, HPE and NVIDIA today launched new AI factory offerings at HPE Discover in Las Vegas. The new lineup includes everything from modular AI… Read Article
Industrial AI isn’t slowing down. Germany is ready. Following London Tech Week and GTC Paris at VivaTech, NVIDIA founder and CEO Jensen Huang’s European tour continued with a stop in… Read Article
At GTC Paris — held alongside VivaTech, Europe’s largest tech event — NVIDIA founder and CEO Jensen Huang delivered a clear message: Europe isn’t just adopting AI — it’s building… Read Article
In a new effort to advance sovereign AI for European public service media, NVIDIA and the European Broadcasting Union (EBU) are working together to give the media industry access to… Read Article
Telecom companies last year spent nearly $295 billion in capital expenditures and over $1 trillion in operating expenditures. These large expenses are due in part to laborious manual processes that… Read Article
Urban populations are expected to double by 2050, which means around 2.5 billion people could be added to urban areas by the middle of the century, driving the need for… Read Article