Technology can’t stop a hurricane in its tracks — but it can make it easier to rebuild in the wake of one.
Following a natural disaster, responders rush to provide aid and restore infrastructure, relying on limited information to make critical decisions about where resources should be deployed.
It can be challenging to analyze these situations from the ground. So San Francisco-based startup CrowdAI has turned to satellite and aerial imagery, extracting insights that help governments and companies send disaster response efforts where they’re most urgently needed.
“Over the last decade, a tremendous amount of satellite and drone data has come online,” said Devaki Raj, founder and CEO of CrowdAI, a member of the NVIDIA Inception virtual accelerator program. “We’re using recent advances in computer vision to unlock that data.”
A few hundred natural disasters occur each year around the globe. That’s a devastating number in terms of human impact, but it’s a fairly small sample size for a machine learning model being trained to assess disaster damage in disparate regions of the world.
While a major earthquake will occur on average around once a month, any given fault line may go a century or more without a strong seismic event. And though a few dozen hurricanes and typhoons strike each year, they cause destruction across vastly different landscapes.
An effective AI for this use case must be specific enough to identify roads and buildings, but generic enough to recognize what a home or road looks like in South Carolina, as well as Sumatra. To achieve this requires finding enough training data, which consists of diverse shots taken both before and after a disaster.
This was once a challenge, but Jigar Doshi, machine learning lead at CrowdAI, says, “as computer vision has matured, we don’t need that many samples of ‘before fire’ and ‘after fire’ to make great models to predict the impacts of disaster.”
In the aftermath of Hurricane Michael last year, CrowdAI worked with telecoms provider WOW! to assess building damage across Panama City, Fla. Analyzing satellite images of the city, provided by NOAA, helped the provider deploy on-the-ground operators to locations most severely hit by the storm and restore service faster.
When unaided by AI, the responders must physically drive around the area and rely on phone calls from customers to determine which regions need assistance — a time-consuming and imprecise process.
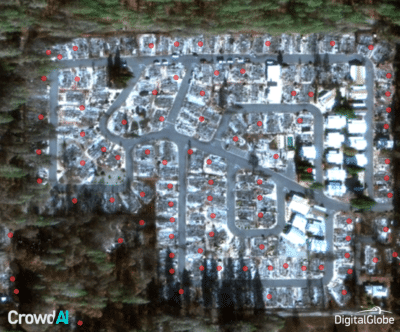
The startup’s research was recognized last year by two of the world’s leading AI conferences: Doshi had a paper accepted at CVPR and presented a joint paper with Facebook at NeurIPS. And after last year’s Camp Fire in Butte County, Calif., the deadliest wildfire in the United States in a century, he and the CrowdAI team used open data to analyze damage.
CrowdAI uses NVIDIA GPUs in the cloud and onsite for training their algorithms and for inference. Depending on the inference environment used, the AI models can return insights with just a one-second lag.
“When a disaster happens, we aren’t training — we’re just doing inference,” said Raj. “That’s why we need this speed.”
CrowdAI is also exploring models that could forecast damage, as well as deep learning tools that go beyond satellite images to integrate wind, precipitation and social media data.
* Main image shows a Florida neighborhood after Hurricane Michael, overlaid with CrowdAI’s categorization of building damage. Analysis courtesy of CrowdAI and imagery courtesy of NOAA’s National Ocean Service.