Seven finalists including both winners of the 2020 Gordon Bell awards used supercomputers to see more clearly atoms, stars and more — all accelerated with NVIDIA technologies.
Their efforts required the traditional number crunching of high performance computing, the latest data science in graph analytics, AI techniques like deep learning or combinations of all of the above.
The Gordon Bell Prize is regarded as a Nobel Prize in the supercomputing community, attracting some of the most ambitious efforts of researchers worldwide.
AI Helps Scale Simulation 1,000x
Winners of the traditional Gordon Bell award collaborated across universities in Beijing, Berkeley and Princeton as well as Lawrence Berkeley National Laboratory (Berkeley Lab). They used a combination of HPC and neural networks they called DeePMDkit to create complex simulations in molecular dynamics, 1,000x faster than previous work while maintaining accuracy.
In one day on the Summit supercomputer at Oak Ridge National Laboratory, they modeled 2.5 nanoseconds in the life of 127.4 million atoms, 100x more than the prior efforts.
Their work aids understanding complex materials and fields with heavy use of molecular modeling like drug discovery. In addition, it demonstrated the power of combining machine learning with physics-based modeling and simulation on future supercomputers.
Atomic-Scale HPC May Spawn New Materials
Among the finalists, a team including members from Berkeley Lab and Stanford optimized the BerkeleyGW application to bust through the complex math needed to calculate atomic forces binding more than 1,000 atoms with 10,986 electrons, about 10x more than prior efforts.
“The idea of working on a system with tens of thousands of electrons was unheard of just 5-10 years ago,” said Jack Deslippe, a principal investigator on the project and the application performance lead at the U.S. National Energy Research Scientific Computing Center.
Their work could pave a way to new materials for better batteries, solar cells and energy harvesters as well as faster semiconductors and quantum computers.
The team used all 27,654 GPUs on the Summit supercomputer to get results in just 10 minutes, thanks to harnessing an estimated 105.9 petaflops of double-precision performance.
Developers are continuing the work, optimizing their code for Perlmutter, a next-generation system using NVIDIA A100 Tensor Core GPUs that sport hardware to accelerate 64-bit floating-point jobs.
Analytics Sifts Text to Fight COVID
Using a form of data mining called graph analytics, a team from Oak Ridge and Georgia Institute of Technology found a way to search for deep connections in medical literature using a dataset they created with 213 million relationships among 18.5 million concepts and papers.
Their DSNAPSHOT (Distributed Accelerated Semiring All-Pairs Shortest Path) algorithm, using the team’s customized CUDA code, ran on 24,576 V100 GPUs on Summit, delivering results on a graph with 4.43 million vertices in 21.3 minutes. They claimed a record for deep search in a biomedical database and showed the way for others.
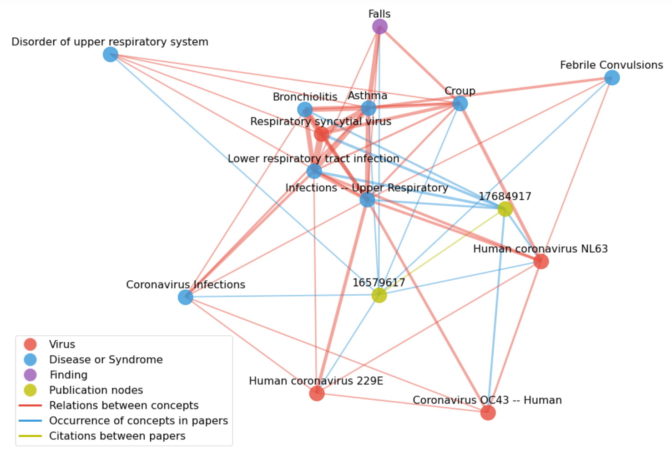
“Looking forward, we believe this novel capability will enable the mining of scholarly knowledge … (and could be used in) natural language processing workflows at scale,” Ramakrishnan Kannan, team lead for computational AI and machine learning at Oak Ridge, said in an article on the lab’s site.
Tuning in to the Stars
Another team pointed the Summit supercomputer at the stars in preparation for one of the biggest big-data projects ever tackled. They created a workflow that handled six hours of simulated output from the Square Kilometer Array (SKA), a network of thousands of radio telescopes expected to come online later this decade.
Researchers from Australia, China and the U.S. analyzed 2.6 petabytes of data on Summit to provide a proof of concept for one of SKA’s key use cases. In the process they revealed critical design factors for future radio telescopes and the supercomputers that study their output.
The team’s work generated 247 GBytes/second of data and spawned 925 GBytes/s in I/O. Like many other finalists, they relied on the fast, low-latency InfiniBand links powered by NVIDIA Mellanox networking, widely used in supercomputers like Summit to speed data among thousands of computing nodes.
Simulating the Coronavirus with HPC+AI
The four teams stand beside three other finalists who used NVIDIA technologies in a competition for a special Gordon Bell Prize for COVID-19.
The winner of that award used all the GPUs on Summit to create the largest, longest and most accurate simulation of a coronavirus to date.
“It was a total game changer for seeing the subtle protein motions that are often the important ones, that’s why we started to run all our simulations on GPUs,” said Lilian Chong, an associate professor of chemistry at the University of Pittsburgh, one of 27 researchers on the team.
“It’s no exaggeration to say what took us literally five years to do with the flu virus, we are now able to do in a few months,” said Rommie Amaro, a researcher at the University of California at San Diego who led the AI-assisted simulation.