Life choices can change a person’s DNA — literally.
Gene changes that occur in human cells over a person’s lifetime, known as somatic mutations, cause the vast majority of cancers. They can be triggered by environmental or behavioral factors such as exposure to ultraviolet light or radiation, drinking or smoking.
By using NVIDIA GPUs to analyze the signature, or molecular fingerprint, of these mutations, researchers can better understand known causes of cancer, discover new risk factors and investigate why certain cancers are more common in certain areas of the world than others.
The Cancer Grand Challenges’ Mutographs team, an international research group funded by Cancer Research U.K., is using NVIDIA GPU-accelerated machine learning models to study DNA from the tumors of 5,000 patients with five cancer types: pancreas, kidney and colorectal cancer, as well as two kinds of esophageal cancer.
Using powerful NVIDIA DGX systems, researchers from the Wellcome Sanger Institute — a world leader in genomics — and the University of California, San Diego, collaborated with NVIDIA developers to achieve more than 30x acceleration when running their machine learning software SigProfiler.
“Research projects such as the Mutographs Grand Challenge are just that — grand challenges that push the boundary of what’s possible,” said Pete Clapham, leader of the Informatics Support Group at the Wellcome Sanger Institute. “NVIDIA DGX systems provide considerable acceleration that enables the Mutographs team to not only meet the project’s computational demands, but to drive it even further, efficiently delivering previously impossible results.”
Molecular Detective Work
Just as every person has a unique fingerprint, cancer-causing somatic mutations have unique patterns that show up in a cell’s DNA.
“At a crime scene, investigators will lift fingerprints and run those through a database to find a match,” said Ludmil Alexandrov, computational lead on the project and an assistant professor of cellular and molecular medicine at UCSD. “Similarly, we can take a molecular fingerprint from cells collected in a patient’s biopsy and see if it matches a risk factor like smoking or ultraviolet light exposure.”
Some somatic mutations have known sources, like those Alexandrov mentions. But the machine learning model can pull out other mutation patterns that occur repeatedly in patients with a specific cancer, but have no known source.
When that happens, Alexandrov teams up with other scientists to test hypotheses and perform large-scale experiments to discover the cancer-causing culprit.
Discovering a new risk factor can help improve cancer prevention. Researchers in 2018 traced back a skin cancer mutational signature to an immunosuppressant drug, which now lists the condition as one of its possible side effects, and helps doctors better monitor patients being treated with the drug.
Enabling Whirlwind Tours of Global Data
In cases where the source of a mutational signature is known, researchers can analyze trends in the occurrence of specific kinds of somatic mutations (and their corresponding cancers) in different regions of the world as well as over time.
“Certain cancers are very common in one part of the world, and very rare in others. And when people migrate from one country to another, they tend to acquire the cancer risk of the country they move to,” said Alexandrov. “What that tells you is that it’s mostly environmental.”
Researchers on the Mutographs project are studying a somatic mutation linked to esophageal cancer, a condition some studies have correlated with the drinking of scalding beverages like tea or maté.
Esophageal cancer is much more common in Eastern South America, East Africa and Central Asia than in North America or West Africa. Finding the environmental or lifestyle factor that puts people at higher risk can help with prevention and early detection of future cases.
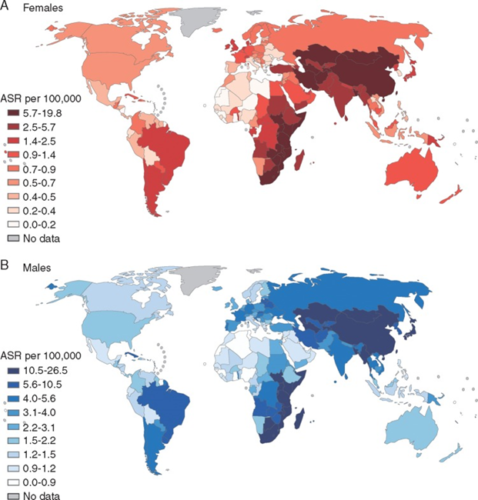
The Mutographs researchers teamed up with NVIDIA to accelerate the most time-consuming parts of the SigProfiler AI framework on NVIDIA GPUs. When running pipeline jobs with double precision on NVIDIA DGX systems, the team observed more than 30x acceleration compared to using CPU hardware. With single precision, Alexandrov says, SigProfiler runs significantly faster, achieving around a 50x speedup.
The DGX system’s optimized software and NVLink interconnect technology also enable the scaling of AI models across all eight NVIDIA V100 Tensor Core GPUs within the system for maximum performance in both model development and deployment.
For research published in Nature this year, Alexandrov’s team analyzed data from more than 20,000 cancer patients, which used to take almost a month.
“With NVIDIA DGX, we can now do that same analysis in less than a day,” he said. “That means we can do much more testing, validation and exploration.”
Subscribe to NVIDIA healthcare news here.
Main image credit: Wellcome Sanger Institute