Look who just set new speed records for training AI models fast: Dell Technologies, Inspur Electronic Information, Supermicro and — in its debut on the MLPerf benchmarks — Azure, all using NVIDIA AI.
Our platform set records across all eight popular workloads in the MLPerf training 1.1 results announced today.
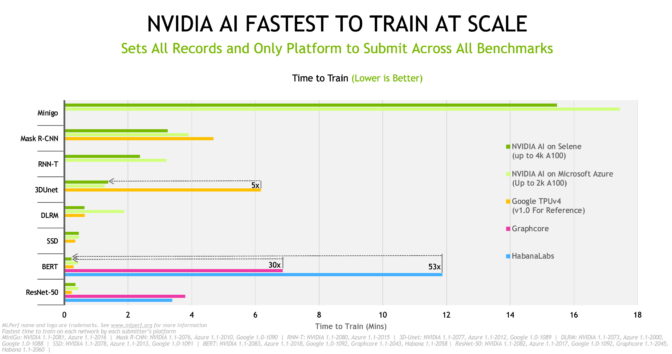
NVIDIA A100 Tensor Core GPUs delivered the best normalized per-chip performance. They scaled with NVIDIA InfiniBand networking and our software stack to deliver the fastest time to train on Selene, our in-house AI supercomputer based on the modular NVIDIA DGX SuperPOD.
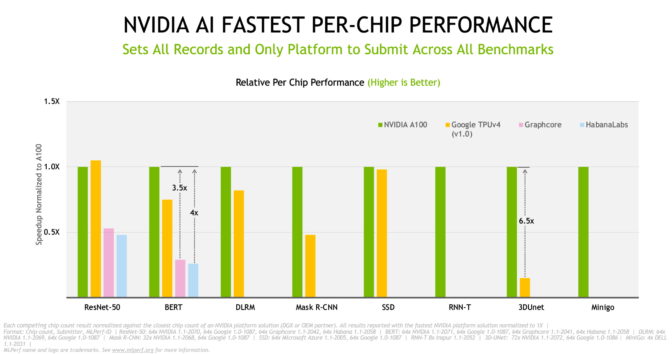
A Cloud Sails to the Top
When it comes to training AI models, Azure’s NDm A100 v4 instance is the fastest on the planet, according to the latest results. It ran every test in the latest round and scaled up to 2,048 A100 GPUs.
Azure showed not only great performance, but great performance that’s available for anyone to rent and use today, in six regions across the U.S.
AI training is a big job that requires big iron. And we want users to train models at record speed with the service or system of their choice.
That’s why we’re enabling NVIDIA AI with products for cloud services, co-location services, corporations and scientific computing centers, too.
Server Makers Flex Their Muscles
Among OEMs, Inspur Electronic Information set the most records in single-node performance with its eight-way GPU systems, the NF5688M6 and the liquid-cooled NF5488A5. Dell and Supermicro set records on four-way A100 GPU systems.
A total of 10 NVIDIA partners submitted results in the round, eight OEMs and two cloud-service providers. They made up more than 90 percent of all submissions.
This is the fifth and strongest showing to date for the NVIDIA ecosystem in training tests from MLPerf.
Our partners do this work because they know MLPerf is the only industry-standard, peer-reviewed benchmark for AI training and inference. It’s a valuable tool for customers evaluating AI platforms and vendors.
Servers Certified for Speed
Baidu PaddlePaddle, Dell Technologies, Fujitsu, GIGABYTE, Hewlett Packard Enterprise, Inspur Electronic Information, Lenovo and Supermicro submitted results in local data centers, running jobs on both single and multiple nodes.
Nearly all our OEM partners ran tests on NVIDIA-Certified Systems, servers we validate for enterprise customers who want accelerated computing.
The range of submissions shows the breadth and maturity of an NVIDIA platform that provides optimal solutions for businesses working at any scale.
Both Fast and Flexible
NVIDIA AI was the only platform participants used to make submissions across all benchmarks and use cases, demonstrating versatility as well as high performance. Systems that are both fast and flexible provide the productivity customers need to speed their work.
The training benchmarks cover eight of today’s most popular AI workloads and scenarios — computer vision, natural language processing, recommendation systems, reinforcement learning and more.
MLPerf’s tests are transparent and objective, so users can rely on the results to make informed buying decisions. The industry benchmarking group, formed in May 2018, is backed by dozens of industry leaders including Alibaba, Arm, Google, Intel and NVIDIA.
20x Speedups in Three Years
Looking back, the numbers show performance gains on our A100 GPUs of over 5x in just the last 18 months. That’s thanks to continuous innovations in software, the lion’s share of our work these days.
NVIDIA’s performance has increased more than 20x since the MLPerf tests debuted three years ago. That massive speedup is a result of the advances we make across our full-stack offering of GPUs, networks, systems and software.
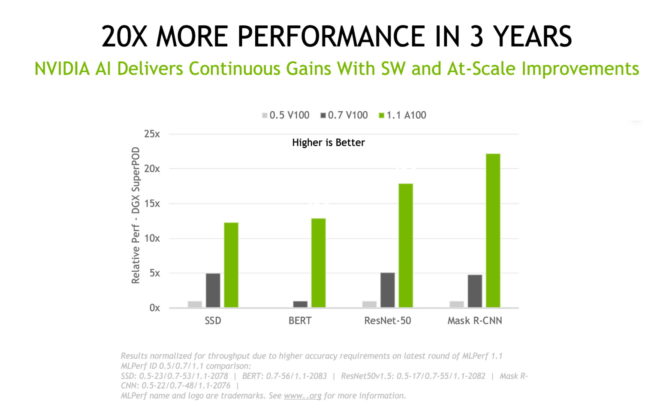
Constantly Improving Software
Our latest advances came from multiple software improvements.
For example, using a new class of memory copy operations, we achieved 2.5x faster operations on the 3D-UNet benchmark for medical imaging.
Thanks to ways you can fine-tune GPUs for parallel processing, we realized a 10 percent speed up on the Mask R-CNN test for object detection and a 27 percent boost for recommender systems. We simply overlapped independent operations, a technique that’s especially powerful for jobs that run across many GPUs.
We expanded our use of CUDA graphs to minimize communication with the host CPU. That brought a 6 percent performance gain on the ResNet-50 benchmark for image classification.
And we implemented two new techniques on NCCL, our library that optimizes communications among GPUs. That accelerated results up to 5 percent on large language models like BERT.
Leverage Our Hard Work
All the software we used is available from the MLPerf repository, so everyone can get our world-class results. We continuously fold these optimizations into containers available on NGC, our software hub for GPU applications.
It’s part of a full-stack platform, proven in the latest industry benchmarks, and available from a variety of partners to tackle real AI jobs today.