You can’t be first if you’re not fast.
Inside the world’s top companies, teams of researchers and data scientists are creating ever more complex AI models, which need to be trained, fast.
That’s why leadership in AI demands leadership in AI infrastructure. And that’s why the AI training results released today by MLPerf matter.
Across all six of six MLPerf categories, NVIDIA demonstrated world-class performance and versatility. Our AI platform set eight records in training performance, including three in overall performance at scale and five on a per-accelerator basis.
Record Type | Benchmark | Record |
---|---|---|
Max Scale (Minutes to Train) | Object Detection (Heavy Weight) - Mask R-CNN | 18.47 mins |
Translation (Recurrent) - GNMT | 1.8 mins | |
Reinforcement Learning - MiniGo | 13.57 mins | |
Per Accelerator (Hours to Train) | Object Detection (Heavy Weight) - Mask R-CNN | 25.39 hrs |
Object Detection (Light Weight) - SSD | 3.04 hrs | |
Translation (Recurrent) - GNMT | 2.63 hrs | |
Translation (Non-recurrent) - Transformer | 2.61 hrs | |
Reinforcement Learning - MiniGo | 3.65 hrs |
Table 1: NVIDIA MLPerf AI Records
Per accelerator comparison derived from reported performance for MLPerf 0.6 on a single NVIDIA DGX-2H (16 V100 GPUs) compared to other submissions at same scale except for MiniGo, where NVIDIA DGX-1 (8 V100 GPUs) submission was used | MLPerf ID Max Scale: Mask R-CNN: 0.6-23, GNMT: 0.6-26, MiniGo: 0.6-11 | MLPerf ID Per Accelerator: Mask R-CNN, SSD, GNMT, Transformer: all use 0.6-20, MiniGo: 0.6-10
These numbers — backed by Google, Intel, Baidu, NVIDIA and the dozens of other top technology companies and universities behind the creation of MLPerf’s suite of AI benchmarks — translate into innovation where it counts.
Simply put, our AI platform now slashes through models that once took a whole workday to train in less than two minutes.
Companies know unlocking that kind of productivity is key. Supercomputers are now the essential instruments of AI, and AI leadership requires strong AI computing infrastructure.
Our latest MLPerf results bring all these strands together, demonstrating the benefits of weaving our NVIDIA V100 Tensor Core GPUs into supercomputing-class infrastructure.
In spring 2017, it took a full workday — eight hours — for an NVIDIA DGX-1 system loaded with V100 GPUs to train the image recognition model ResNet-50.
Today an NVIDIA DGX SuperPOD — using the same V100 GPUs, now interconnected with Mellanox InfiniBand and the latest NVIDIA-optimized AI software for distributed AI training — completed the task in just 80 seconds.
That’s less time than it takes to get a cup of coffee.
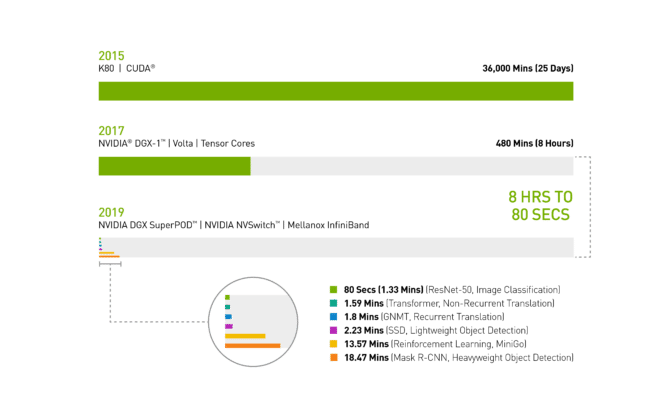
2019 MLPerf ID (in order from top to bottom of chart): ResNet-50: 0.6-30 | Transformer: 0.6-28 | GNMT: 0.6-14 | SSD: 0.6-27 | MiniGo: 0.6-11 | Mask R-CNN: 0.6-23
The Essential Instrument of AI: DGX SuperPOD Masters Workloads Faster
A close look at today’s MLPerf results shows the NVIDIA DGX SuperPOD is the only AI platform able to complete each of the six MLPerf categories in less than 20 minutes:
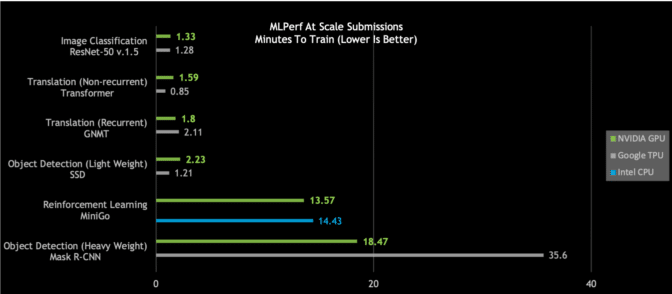
An even closer look reveals NVIDIA’s AI platform stands out on the hardest AI problems as measured by total time to train: heavyweight object detection and reinforcement learning.
Heavyweight object detection using the Mask R-CNN deep neural network provides users with advanced instance segmentation. Its uses include combining it with multiple data sources — cameras, sensors, lidar, ultrasound and more — to precisely identify and locate specific objects.
This type of AI workload helps train autonomous vehicles, providing precise locations of pedestrians and other objects to self-driving cars. Another real-life application helps doctors find and identify tumors in medical scans. Critical stuff.
NVIDIA’s heavyweight object detection submission, which came in at just under 19 minutes, delivers nearly twice the performance as the next best submission.
Reinforcement learning is another difficult category. This AI method trains robots working on factory floors to streamline production. It’s also used in cities to control traffic lights to reduce congestion. Using an NVIDIA DGX SuperPOD, NVIDIA trained the MiniGo AI reinforcement training model in a record-setting 13.57 minutes.
No More Time for Coffee: Instant AI Infrastructure Delivers World-Leading Performance
Speeding innovation, however, is about more than beating benchmarks. That’s why we made DGX SuperPOD not only powerful, but easy to set up.
Fully configured with optimized CUDA-X AI software freely available from our NGC container registry, DGX SuperPODs deliver world-leading AI performance out of the box.
They plug into an ecosystem of more than 1.3 million CUDA developers we work with to support every AI framework and development environment.
We’ve helped optimize millions of lines of code so our customers can bring their AI projects to life everywhere you can find NVIDIA GPUs: on the cloud, in data centers and at the edge.
AI Infrastructure That’s Fast Now, Faster Tomorrow
Better still, this is a platform that’s always getting faster. We publish new optimizations and performance improvements to CUDA-X AI software every month, with integrated software stacks freely available for download on our NGC container registry. That includes containerized frameworks, pre-trained models and scripts.
With such innovation to the CUDA-X AI software stack, an NVIDIA DGX-2H server gained up to 80 percent more throughput on our MLPerf 0.6 submissions than what we posted just seven months ago.
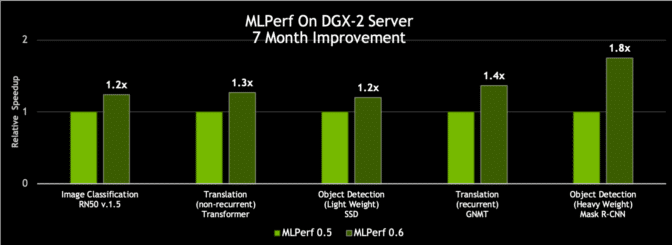
Add it up and these efforts represent an investment of tens of billions of dollars. All so you can get your work done fast today. And faster tomorrow.
Dive deeper into the performance results in NVIDIA Developer Blog. And check out the infographic.