NVIDIA’s partners are delivering GPU-accelerated systems that train AI models faster than anyone on the planet, according to the latest MLPerf results released today.
Seven companies put at least a dozen commercially available systems, the majority NVIDIA-Certified, to the test in the industry benchmarks. Dell, Fujitsu, GIGABYTE, Inspur Electronic Information, Lenovo, Nettrix and Supermicro joined NVIDIA to demonstrate industry-leading results training neural networks with NVIDIA A100 Tensor Core GPUs.
Only NVIDIA and its partners ran all eight workloads in the latest round of benchmarks. Together, our work made up more than three-quarters of all submissions, and the results were stunning.
Compared to last year’s scores, we delivered up to 3.5x more performance. For massive jobs that demand the most muscle, we mustered resources from a record 4,096 GPUs, more than any other submission.
Why MLPerf Matters
This is the fourth and strongest showing for the NVIDIA ecosystem in training tests from MLPerf, an industry benchmarking group formed in May 2018.
MLPerf gives users the confidence to make informed buying decisions. It’s backed by dozens of industry leaders including Alibaba, Arm, Baidu, Google, Intel and NVIDIA, so the tests are transparent and objective.
The benchmarks are based on today’s most popular AI workloads and scenarios, covering computer vision, natural-language processing, recommendation systems, reinforcement learning and more. And the training benchmarks focus on what users care about most — time to train a new AI model.
Speed + Flexibility = Productivity
Ultimately, the return on a customer’s infrastructure investment depends on their productivity. That comes from an ability to be both fast and flexible when running these many kinds of AI workloads.
That’s why users need flexible, yet powerful systems that can get a variety of AI models into production fast, speeding time to market and maximizing the productivity of their valuable data science teams.
In the latest MLPerf results, the NVIDIA AI platform set performance records by training models in the shortest time across all eight benchmarks in the commercially available submissions category.
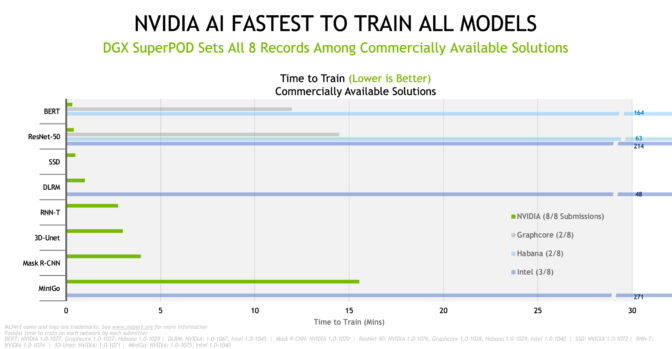
We ran the at-scale tests on Selene, the fastest commercial AI supercomputer in the world, according to the latest TOP500 rankings. It’s based on the same NVIDIA DGX SuperPOD architecture that powers a dozen other systems on the list.
The ability to scale to large clusters is the toughest challenge in AI and one of our core strengths.
In chip-to-chip comparisons, NVIDIA and its partners set records across all eight benchmarks in the latest tests on commercially available systems.
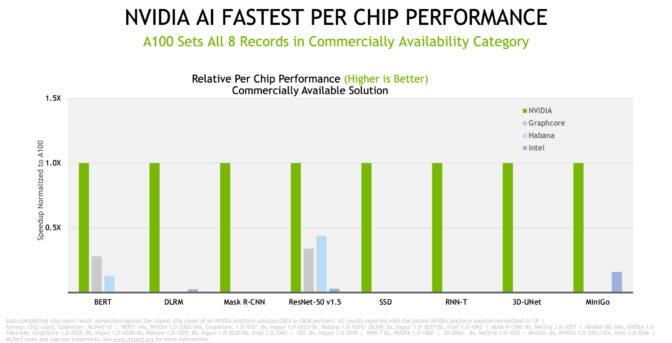
Overall, the results below show our performance rose up to 6.5x in 2.5 years, a testament to work across the full-stack NVIDIA platform of GPUs, systems and software.
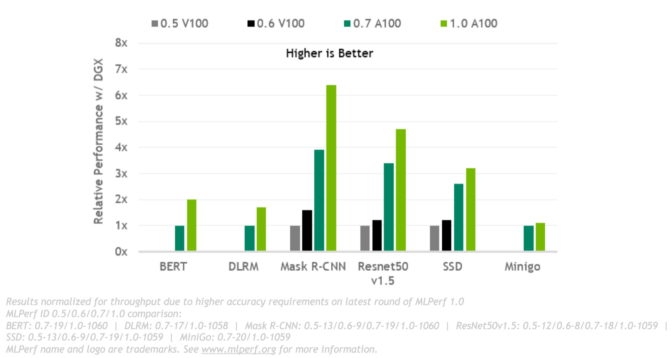
Broad Ecosystem Offers Best Value, Choice
The MLPerf results demonstrate performance across a variety of NVIDIA-based AI platforms with plenty of new and innovative systems. They span entry-level edge servers to AI supercomputers that accommodate thousands of GPUs.
The seven partners participating in the latest benchmarks are among nearly two dozen cloud-service providers and OEMs with products or plans for online instances, servers and PCIe cards using NVIDIA A100 GPUs, including nearly 40 NVIDIA-Certified Systems.
Our ecosystem offers customers choices in a wide range of deployment models — from instances that are rentable by the minute to on-prem servers and managed services — providing the most value per dollar in the industry.
Results across all the MLPerf tests show our performance keeps rising over time. That comes from a platform with software that’s mature and constantly improving, so teams can get started fast with systems that keep getting better.
How We Did It
It’s the second round of MLPerf tests for our A100 GPUs. Speedups came from advances detailed in a separate article that spanned GPUs, systems, networking and AI software.
For example, our engineers found a way to launch full neural network models using CUDA Graphs, a software package of NVIDIA CUDA operations and their dependencies. That eliminated CPU bottlenecks in past tests that released AI models as a chain of many individual components, called kernels.
In addition, tests at scale used NVIDIA SHARP, software that consolidates multiple communications jobs inside a network switch, reducing network traffic and time waiting for a CPU.
The combination of the CUDA Graphs and SHARP allowed training jobs in the data center to access a record number of GPUs. It’s the muscle needed in many areas such as natural-language processing, where AI models are growing to include billions of parameters.
Other gains came from expanded memory on the latest A100 GPUs that increase memory bandwidth nearly 30 percent to more than 2 TB/s.
Customers Care About MLPerf
A wide variety of AI users find these benchmarks useful.
“The MLPerf benchmark provides a transparent apples-to-apples comparison across multiple AI platforms to showcase actual performance in diverse real-world use cases,” said a spokesman for Sweden’s Chalmers University, which conducts research across areas from nanotechnology to climate studies.
The benchmarks help users find the AI products that meet the requirements of some of the world’s largest and most advanced factories. For example, TSMC, a global leader in chip manufacturing, uses machine learning to improve optical proximity correction (OPC) and etch simulation.
“To fully realize the potential of machine learning in model training and inference, we’re working with the NVIDIA engineering team to port our Maxwell simulation and inverse lithography technology engine to GPUs and see very significant speedups. The MLPerf benchmark is an important factor in our decision making,” said Danping Peng, director of TSMC’s OPC department.
Traction in Medicine, Manufacturing
The benchmarks also are useful for researchers pushing the limits of AI to improve healthcare.
“We’ve worked closely with NVIDIA to bring innovations like 3DUNet to the healthcare market. Industry-standard MLPerf benchmarks provide relevant performance data to the benefit of IT organizations and developers to get the right solution to accelerate their specific projects and applications,” said Klaus Maier-Hein, head of medical image computing at DKFZ, the German cancer research center.
A world leader in research and manufacturing, Samsung also uses MLPerf benchmarks as it implements AI to boost product performance and manufacturing productivity.
“Productizing these AI advances requires us to have the best computing platform available. The MLPerf benchmark streamlines our selection process by providing us with an open, direct evaluation method to assess uniformly across platform vendors,” said a spokesperson for Samsung Electronics.
Get These Same Results, Tools
All the software we used for the latest submissions is available from the MLPerf repository, so anyone can reproduce our benchmark results. We continually add this code into our deep learning frameworks and containers available on NGC, our software hub for GPU applications.
It’s part of a full-stack AI platform, proven in the latest industry benchmarks, and available from a variety of partners to tackle real AI jobs today.