NVIDIA delivers the world’s fastest AI training performance among commercially available products, according to MLPerf benchmarks released today.
The A100 Tensor Core GPU demonstrated the fastest performance per accelerator on all eight MLPerf benchmarks. For overall fastest time to solution at scale, the DGX SuperPOD system, a massive cluster of DGX A100 systems connected with HDR InfiniBand, also set eight new performance milestones. The real winners are customers applying this performance today to transform their businesses faster and more cost effectively with AI.
This is the third consecutive and strongest showing for NVIDIA in training tests from MLPerf, an industry benchmarking group formed in May 2018. NVIDIA set six records in the first MLPerf training benchmarks in December 2018 and eight in July 2019.
NVIDIA set records in the category customers care about most: commercially available products. We ran tests using our latest NVIDIA Ampere architecture as well as our Volta architecture.
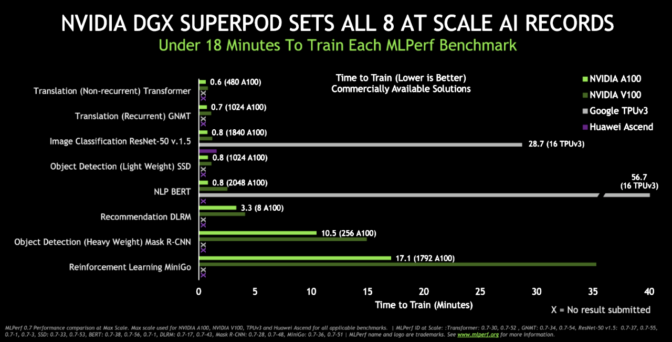
NVIDIA was the only company to field commercially available products for all the tests. Most other submissions used the preview category for products that may not be available for several months or the research category for products not expected to be available for some time.
NVIDIA Ampere Ramps Up in Record Time
In addition to breaking performance records, the A100, the first processor based on the NVIDIA Ampere architecture, hit the market faster than any previous NVIDIA GPU. At launch, it powered NVIDIA’s third-generation DGX systems, and it became publicly available in a Google cloud service just six weeks later.
Also helping meet the strong demand for A100 are the world’s leading cloud providers, such as Amazon Web Services, Baidu Cloud, Microsoft Azure and Tencent Cloud, as well as dozens of major server makers, including Dell Technologies, Hewlett Packard Enterprise, Inspur Electronic Information and Supermicro.
Users across the globe are applying the A100 to tackle the most complex challenges in AI, data science and scientific computing.
Some are enabling a new wave of recommendation systems or conversational AI applications while others power the quest for treatments for COVID-19. All are enjoying the greatest generational performance leap in eight generations of NVIDIA GPUs.
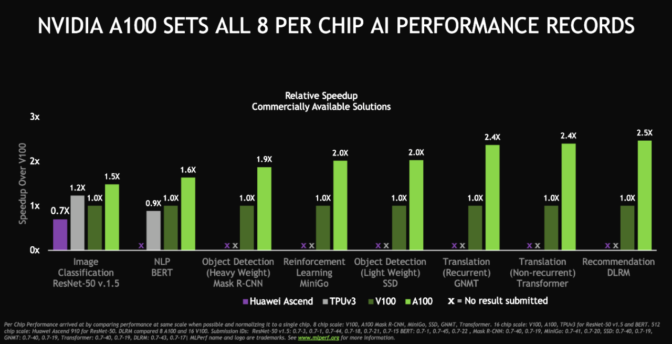
A 4x Performance Gain in 1.5 Years
The latest results demonstrate NVIDIA’s focus on continuously evolving an AI platform that spans processors, networking, software and systems.
For example, the tests show at equivalent throughput rates today’s DGX A100 system delivers up to 4x the performance of the system that used V100 GPUs in the first round of MLPerf training tests. Meanwhile, the original DGX-1 system based on NVIDIA V100 can now deliver up to 2x higher performance thanks to the latest software optimizations.
These gains came in less than two years from innovations across the AI platform. Today’s NVIDIA A100 GPUs — coupled with software updates for CUDA-X libraries — power expanding clusters built with Mellanox HDR 200Gb/s InfiniBand networking.
HDR InfiniBand enables extremely low latencies and high data throughput, while offering smart deep learning computing acceleration engines via the scalable hierarchical aggregation and reduction protocol (SHARP) technology.
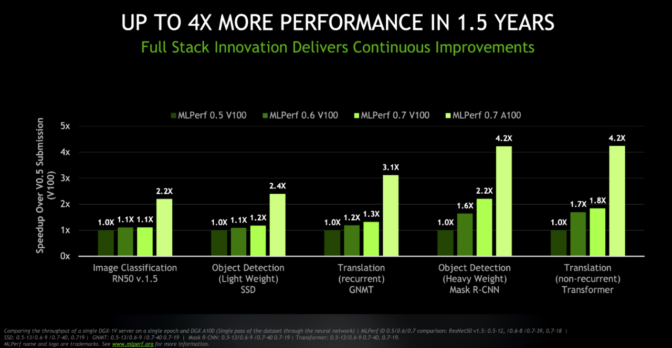
NVIDIA Shines in Recommendation Systems, Conversational AI, Reinforcement Learning
The MLPerf benchmarks — backed by organizations including Amazon, Baidu, Facebook, Google, Harvard, Intel, Microsoft and Stanford — constantly evolve to remain relevant as AI itself evolves.
The latest benchmarks featured two new tests and one substantially revised test, all of which NVIDIA excelled in. One ranked performance in recommendation systems, an increasingly popular AI task; another tested conversational AI using BERT, one of the most complex neural network models in use today. Finally, the reinforcement learning test used Mini-go with the full-size 19×19 Go board and was the most complex test in this round involving diverse operations from game play to training.
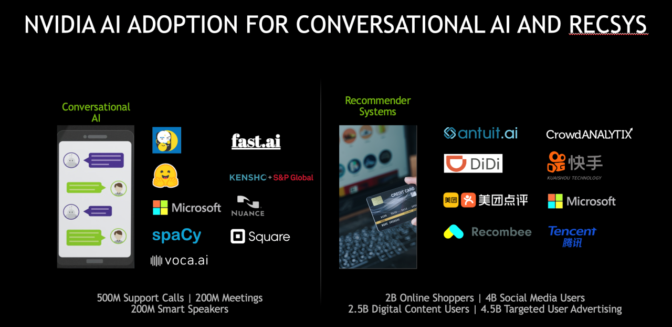
Companies are already reaping the benefits of this performance on these strategic applications of AI.
Alibaba hit a $38 billion sales record on Singles Day in November, using NVIDIA GPUs to deliver more than 100x more queries/second on its recommendation systems than CPUs. For its part, conversational AI is becoming the talk of the town, driving business results in industries from finance to healthcare.
NVIDIA is delivering both the performance needed to run these powerful jobs and the ease of use to embrace them.
Software Paves Strategic Paths to AI
In May, NVIDIA announced two application frameworks, Jarvis (since renamed NVIDIA Riva) for conversational AI and NVIDIA Merlin for recommendation systems. Merlin includes the HugeCTR framework for training that powered the latest MLPerf results.
These are part of a growing family of application frameworks for markets including automotive (NVIDIA DRIVE), healthcare (Clara), robotics (Isaac) and retail/smart cities (Metropolis).
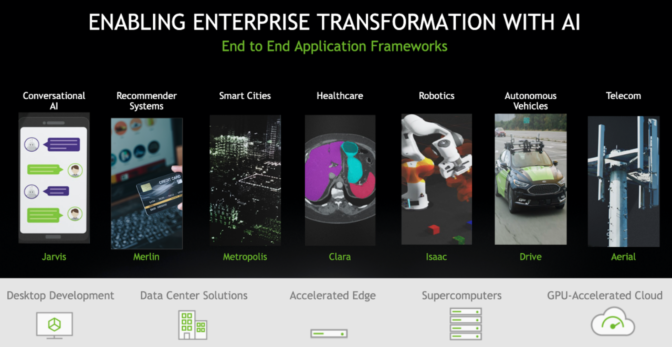
DGX SuperPOD Architecture Delivers Speed at Scale
NVIDIA ran MLPerf tests for systems on Selene, an internal cluster based on the DGX SuperPOD, its public reference architecture for large-scale GPU clusters that can be deployed in weeks. That architecture extends the design principles and best practices used in the DGX POD to serve the most challenging problems in AI today.
Selene recently debuted on the TOP500 list as the fastest industrial system in the U.S. with more than an exaflops of AI performance. It’s also the world’s second most power-efficient system on the Green500 list.
Customers are already using these reference architectures to build DGX PODs and DGX SuperPODs of their own. They include HiPerGator, the fastest academic AI supercomputer in the U.S., which the University of Florida will feature as the cornerstone of its cross-curriculum AI initiative.
Meanwhile, a top supercomputing center, Argonne National Laboratory, is using DGX A100 to find ways to fight COVID-19. Argonne was the first of a half-dozen high performance computing centers to adopt A100 GPUs.
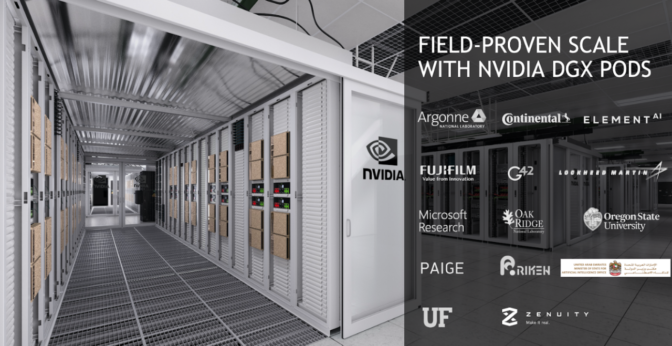
DGX SuperPODs are already driving business results for companies like Continental in automotive, Lockheed Martin in aerospace and Microsoft in cloud-computing services.
These systems are all up and running thanks in part to a broad ecosystem supporting NVIDIA GPUs and DGX systems.
Strong MLPerf Showing by NVIDIA Ecosystem
Of the nine companies submitting results, seven submitted with NVIDIA GPUs including cloud service providers (Alibaba Cloud, Google Cloud and Tencent Cloud) and server makers (Dell, Fujitsu and Inspur Electronic Information), highlighting the strength of NVIDIA’s ecosystem.
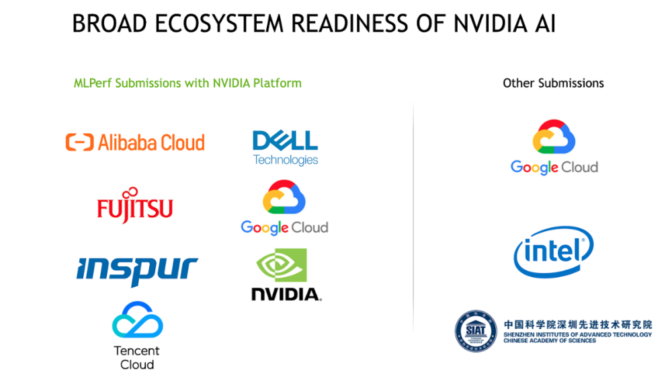
Many of these partners used containers on NGC, NVIDIA’s software hub, along with publicly available frameworks for their submissions.
The MLPerf partners represent part of an ecosystem of nearly two dozen cloud-service providers and OEMs with products or plans for online instances, servers and PCIe cards using NVIDIA A100 GPUs.
Test-Proven Software Available on NGC Today
Much of the same software NVIDIA and its partners used for the latest MLPerf benchmarks is available to customers today on NGC.
NGC is host to several GPU-optimized containers, software scripts, pre-trained models and SDKs. They empower data scientists and developers to accelerate their AI workflows across popular frameworks such as TensorFlow and PyTorch.
Organizations are embracing containers to save time getting to business results that matter. In the end, that’s the most important benchmark of all.
Artist’s rendering at top: NVIDIA’s new DGX SuperPOD, built in less than a month and featuring more than 2,000 NVIDIA A100 GPUs, swept every MLPerf benchmark category for at-scale performance among commercially available products.