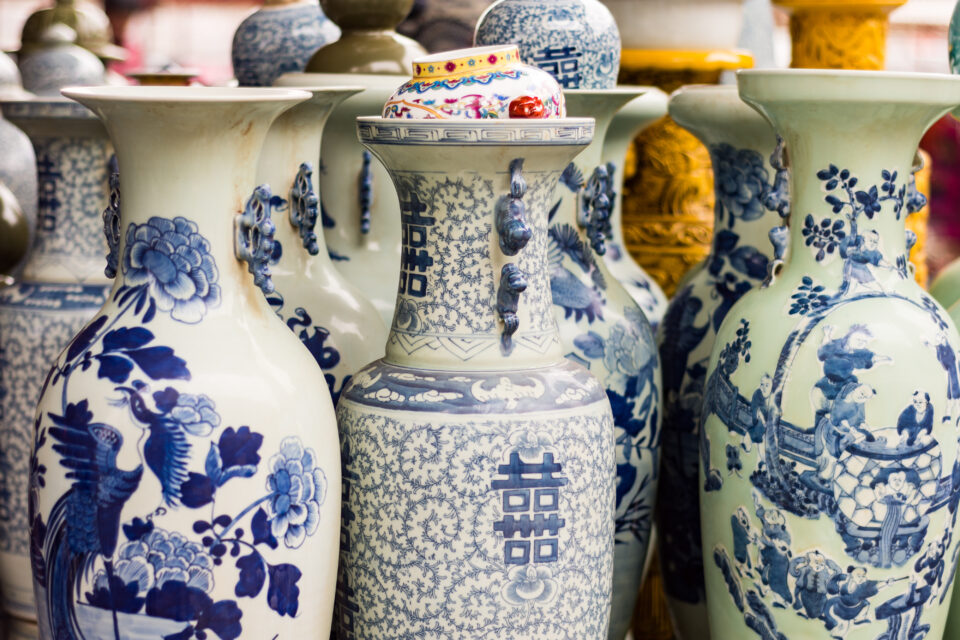
Ceramics — the humble mix of earth, fire and artistry — have been part of a global conversation… Read Article
Ceramics — the humble mix of earth, fire and artistry — have been part of a global conversation… Read Article
At GTC Paris — held alongside VivaTech, Europe’s largest tech event — NVIDIA founder and CEO Jensen Huang delivered a clear message: Europe isn’t just adopting AI — it’s building… Read Article
Autonomous vehicle (AV) stacks are evolving from many distinct models to a unified, end-to-end architecture that executes driving actions directly from sensor data. This transition to using larger models is… Read Article
The roots of many of NVIDIA’s landmark innovations — the foundational technology that powers AI, accelerated computing, real-time ray tracing and seamlessly connected data centers — can be found in… Read Article
American Sign Language is the third most prevalent language in the United States — but there are vastly fewer AI tools developed with ASL data than data representing the country’s… Read Article
Scientists everywhere can now access Evo 2, a powerful new foundation model that understands the genetic code for all domains of life. Unveiled today as the largest publicly available AI… Read Article
Editor’s note: This article, originally published on March 13, 2023, has been updated. The mics were live and tape was rolling in the studio where the Miles Davis Quintet was… Read Article
AI-driven medicine could deliver life-saving snakebite treatments to the world’s most vulnerable…. Read Article
Editor’s note: This article, originally published on Nov. 15, 2023, has been updated. To understand the latest advancements in generative AI, imagine a courtroom. Judges hear and decide cases based… Read Article