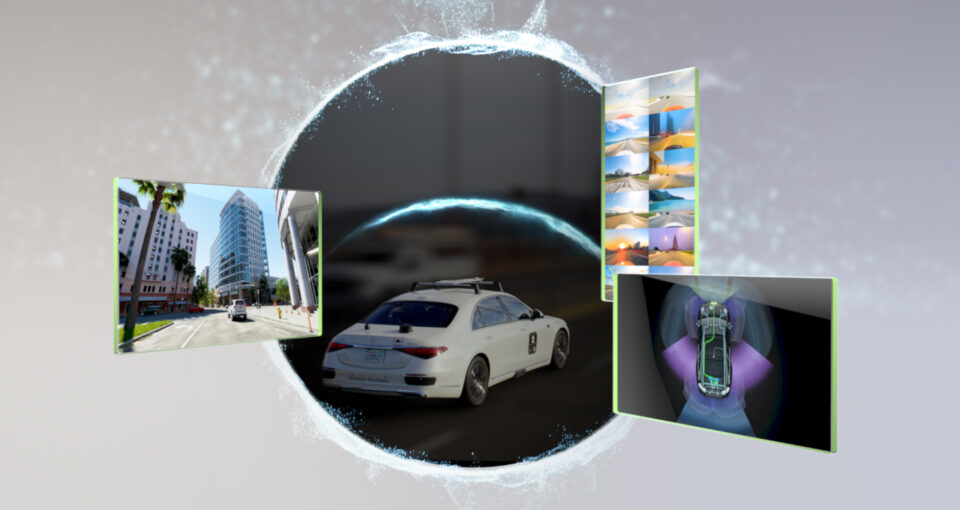
Simulated driving environments enable engineers to safely and efficiently train, test and validate autonomous vehicles (AVs) across countless… Read Article
Simulated driving environments enable engineers to safely and efficiently train, test and validate autonomous vehicles (AVs) across countless… Read Article
In the face of growing labor shortages and need for sustainability, European manufacturers are racing to reinvent their processes to become software-defined and AI-driven. To achieve this, robot developers and… Read Article
NVIDIA was today named an Autonomous Grand Challenge winner at the Computer Vision and Pattern Recognition (CVPR) conference, held this week in Nashville, Tennessee. The announcement was made at the… Read Article
NVIDIA is launching a comprehensive, industry-defining autonomous vehicle (AV) software platform to accelerate large-scale deployment of safe, intelligent transportation innovations for automakers, truck manufacturers, robotaxi companies and startups worldwide. Announced… Read Article
Autonomous vehicle (AV) stacks are evolving from many distinct models to a unified, end-to-end architecture that executes driving actions directly from sensor data. This transition to using larger models is… Read Article
Germany is building on a long history of engineering innovation with new AI investments poised to transform the country’s economy — including the automotive, banking, manufacturing and robotics industries. The… Read Article
The autonomous vehicle (AV) revolution is here — and NVIDIA is at its forefront, bringing more than two decades of automotive computing, software and safety expertise to power innovation from… Read Article
Teaching autonomous robots and vehicles how to interact with the physical world requires vast amounts of high-quality data. To give researchers and developers a head start, NVIDIA is releasing a… Read Article
Physical AI is unlocking new possibilities at the intersection of autonomy and robotics — accelerating, in particular, the development of autonomous vehicles (AVs). The right technology and frameworks are crucial… Read Article