AI is the most impactful technological advance of our time, transforming every aspect of the global economy.
Five waves of growth have carried AI from inception to ubiquity: the big bang of AI, cloud services, enterprise AI, edge AI and autonomy.
Like other technical breakthroughs — such as industrial machinery, transistors, the internet and mobile computing — AI was conceived in academia and commercialized in successive phases. It first took hold in large, well-resourced organizations before spreading over years to smaller organizations, professionals and consumers.
Since the term “AI” was coined at Dartmouth University in 1956, people in this field have explored many approaches to solving the world’s toughest problems. One of the most popular, deep learning, exploits data structures called neural networks that mirror how human brain cells operate.
Data scientists using deep learning configure a neural network with the parameters that work best for a particular problem, and then feed the AI up to millions of sample questions and answers. With each sample answer, the AI adjusts its neural weights until it can answer the questions on its own — even new ones it hasn’t seen before.
Learn more about the five waves of modern AI, determine which wave your organization is in and gear up for what comes next.
The Big Bang of AI
The first wave of AI computing was its “big bang,” which started with the discovery of deep neural networks.
Three fundamental factors fueled this explosion: academic breakthroughs in deep learning, the widespread availability of big data, and the novel application of GPUs to accelerate deep learning development and training.
Where computer scientists used to specify each AI instruction, algorithms can now write other algorithms, software can write software, and computers can learn on their own. This marked the beginning of the machine learning era.
And over the last decade, deep learning has migrated from academia to commerce, carried by the next four waves of growth.
The Cloud
The first businesses to use AI were large tech companies with the scientific know-how and computing resources to adapt neural networks to benefit their customers. They did so using the cloud — the second wave of AI computing.
Google, for example, applied deep learning to natural language processing to offer Google Translate. Facebook applied AI to identify consumer goods from images to make them shoppable. Through these types of cloud applications, Google, Amazon and Microsoft introduced many of AI’s first real-world applications.
Soon, these large tech companies created infrastructure-as-a-service platforms, unleashing the power of public clouds for enterprises and startups alike, and driving AI adoption further.
Now, companies of all sizes rely on the cloud to get started with AI quickly and affordably. It offers an easy onramp for companies to deploy AI, allowing them to focus on developing and training models, instead of building underlying infrastructure.
Enterprise AI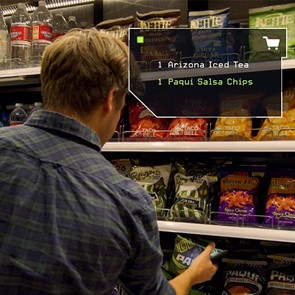
As tools are developed to make AI more accessible, large enterprises are embracing the technology to improve the quality, safety and efficiency of their workflows — and leading the third wave of AI computing. Data scientists in finance, healthcare, environmental services, retail, entertainment and other industries started training neural networks in their own data centers or the cloud.
For example, conversational AI chatbots enhance call centers, and fraud-detection AI monitors unusual activity in online marketplaces. Computer vision acts as a virtual assistant for mechanics, doctors and pilots, providing them with information to make more accurate decisions.
While this wave of AI computing has widespread applications and garners headlines each week, it’s just getting started. Companies are investing heavily in data scientists who can prepare data to train models and machine learning engineers who can create and automate AI training and deployment pipelines.
The Edge
The fourth wave pushes AI from the cloud or data center to the edge, to places like factories, hospitals, airports, stores, restaurants and power grids. The advent of 5G is furthering the ability for edge computing devices to be deployed and managed anywhere. It’s created an explosive opportunity for AI to transform workplaces and for enterprises to realize the value of data from their end users.
With the adoption of IoT devices and advances in compute infrastructure, the proliferation of big data allows enterprises to create and train AI models to be deployed at the edge, where end users are located.
This wave requires machine learning engineers and data scientists to consider the design constraints of AI inference at the edge. Such limits include connectivity, storage, battery power, compute power and physical access for maintenance. Designs must also align with the needs of business owners, IT teams and security operations to better ensure the success of deployments.
Edge AI is also in its early days, but already used across many industries. Computer vision monitors factory floors for safety infractions, scans medical images for anomalous growths and drives cars safely down the freeway. The potential for new applications is limitless.
Autonomy
The fifth wave of AI will be the rise of autonomy — the evolution of AI to the point where AI navigates mobile machinery without human intervention. Cars, trucks, ships, planes, drones and other robots will operate without human piloting. For this to unfold, the network connectivity of 5G, the power of accelerated computing, and continued innovation in the capabilities of neural networks are necessary.
Autonomous AI is making headway, driven by the pandemic, global supply chain constraints and the related need for automation for efficiency in business processes.
Incorporating domains of engineering beyond deep learning, autonomous AI requires machine learning engineers to collaborate with robotics engineers. Together, they work to fulfill the four pillars of a robotics system workflow: collecting and generating ground-truth data, creating the AI model, simulating with a digital twin and operating the robot in the real world.
For robotics, simulation capabilities are especially important in modeling and testing all possible corner cases to mitigate the safety risks of deploying robots in the real world.
Autonomous machines also face novel challenges around deployment, management and security that require coordination across teams in engineering, operations, manufacturing, networking, security and compliance.
Getting Started With AI
Starting with the big bang of AI, the industry has grown quickly and spawned further waves of computing, including cloud services, enterprise AI, edge AI and autonomous machines. These advancements are carrying AI from laboratories to living rooms, improving businesses and the daily lives of consumers.
NVIDIA has spent decades building the computational products and software necessary to enable the AI ecosystem to drive these waves of growth. In addition to developing and implementing AI into the company, NVIDIA has helped countless enterprises, startups, factories, healthcare firms and more to adopt, implement and scale their own AI initiatives.
Whether starting an initial AI project, transitioning a team into AI workloads or looking at infrastructure blueprints and expansions, set your AI projects up for success.