Two revolutions are meeting in the field of life sciences — the explosion of digital data and the rise of AI computing to help healthcare professionals make sense of it all, said Daphne Koller and Kimberly Powell at this week’s GPU Technology Conference,.
Powell, NVIDIA’s vice president of healthcare, presented an overview of AI innovation in medicine that highlighted advances in drug discovery, medical imaging, genomics and intelligent medical instruments.
“There’s a digital biology revolution underway, and it’s generating enormous data, far too complex for human understanding,” she said. “With algorithms and computations at the ready, we now have the third ingredient — data — to truly enter the AI healthcare era.”
And Koller, a Stanford adjunct professor and CEO of the AI drug discovery company Insitro, focused on AI solutions in her talk outlining the challenges of drug development and the ways in which predictive machine learning models can enable a better understanding of disease-related biological data.
Digital biology “allows us to measure biological systems in entirely new ways, interpret what we’re measuring using data science and machine learning, and then bring that back to engineer biology to do things that we’d never otherwise be able to do,” she said.
Watch replays of these talks — part of a packed lineup of more than 100 healthcare sessions among 1,600 on-demand sessions — by registering free for GTC through April 23. Registration isn’t required to watch a replay of the keynote address by NVIDIA CEO Jensen Huang.
Data-Driven Insights into Disease
Recent advancements in biotechnology — including CRISPR, induced pluripotent stem cells and more widespread availability of DNA sequencing — have allowed scientists to gather “mountains of data,” Koller said in her talk, “leaving us with a problem of how to interpret those data.”
“Fortunately, this is where the other revolution comes in, which is that using machine learning to interpret and identify patterns in very large amounts of data has transformed virtually every sector of our existence,” she said.
The data-intensive process of drug discovery requires researchers to understand the biological structure of a disease, and then vet potential compounds that could be used to bind with a critical protein along the disease pathway. Finding a promising therapeutic is a complex optimization problem, and despite the exponential rise in the amount of digital data available in the last decade or two, the process has been getting slower and more expensive.
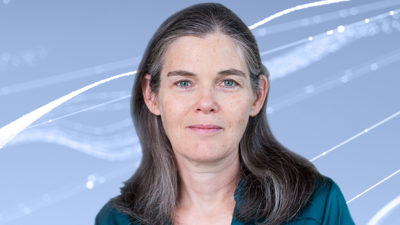
Known as Eroom’s law, this observation finds that the research and development cost for bringing a new drug to market has trended upward since the 1980s, taking pharmaceutical companies more time and money. Koller says that’s because of all the potential drug candidates that fail to get approved for use.
“What we aim to do at Insitro is to understand those failures, and try and see whether machine learning — combined with the right kind of data generation — can get us to make better decisions along the path and avoid a lot of those failures,” she said. “Machine learning is able to see things that people just cannot see.”
Bringing AI to vast datasets can help scientists determine how physical characteristics like height and weight, known as phenotypes, relate to genetic variants, known as genotypes. In many cases, “these associations give us a hint about the causal drivers of disease,” said Koller.
She gave the example of NASH, or nonalcoholic steatohepatitis, a common liver condition related to obesity and diabetes. To study underlying causes and potential treatments for NASH, Insitro worked with biopharmaceutical company Gilead to apply machine learning to liver biopsy and RNA sequencing data from clinical trial data representing hundreds of patients.
The team created a machine learning model to analyze biopsy images to capture a quantitative representation of a patient’s disease state, and found even with just a weak level of supervision, the AI’s predictions aligned with the scores assigned by clinical pathologists. The models could even differentiate between images with and without NASH, which is difficult to determine with the naked eye.
Accelerating the AI Healthcare Era
It’s not enough to just have abundant data to create an effective deep learning model for medicine, however. Powell’s GTC talk focused on domain-specific computational platforms — like the NVIDIA Clara application framework for healthcare — that are tailored to the needs and quirks of medical datasets.
The NVIDIA Clara Discovery suite of AI libraries harnesses transformer models, popular in natural language processing, to parse biomedical deta. Using the NVIDIA Megatron framework for training transformers helps researchers build models with billions of parameters — like MegaMolBart, an NLP generative drug discovery model in development by NVIDIA and AstraZeneca for use in reaction prediction, molecular optimization and de novo molecular generation.
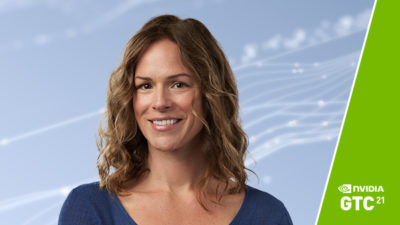
University of Florida Health has also used the NVIDIA Megatron framework and NVIDIA BioMegatron pre-trained model to develop GatorTron, the largest clinical language model to date, which was trained on more than 2 million patient records with more than 50 million interactions.
“With biomedical data at scale of petabytes, and learning at the scale of billions and soon trillions of parameters, transformers are helping us do and find the unexpected,” Powell said.
Clinical decisions, too, can be supported by AI insights that parse data from health records, medical imaging instruments, lab tests, patient monitors and surgical procedures.
“No one hospital’s the same, and no healthcare practice is the same,” Powell said. “So we need an entire ecosystem approach to developing algorithms that can predict the future, see the unseen, and help healthcare providers make complex decisions.”
The NVIDIA Clara framework has more than 40 domain-specific pretrained models available in the NGC catalog — including NVIDIA Federated Learning, which allows different institutions to collaborate on AI model development without sharing patient data with each other, overcoming challenges of data governance and privacy.
And to power the next generation of intelligent medical instruments, the newly available NVIDIA Clara AGX developer kit helps hospitals develop and deploy AI across smart sensors such as endoscopes, ultrasound devices and microscopes.
“As sensor technology continues to innovate, so must the computing platforms that process them,” Powell said. “With AI, instruments can become smaller, cheaper and guide an inexperienced user through the acquisition process.”
These AI-driven devices could help reach areas of the world that lack access to many medical diagnostics today, she said. “The instruments that measure biology, see inside our bodies and perform surgeries are becoming intelligent sensors with AI and computing.”
GTC registration is open through April 23. Attendees will have access to on-demand content through May 11. For more, subscribe to NVIDIA healthcare news, and follow NVIDIA Healthcare on Twitter.