You don’t need a hunky sea god with a three-pronged spear to make AI work, but a growing group of companies from car makers to cloud service providers say you’ll feel a sea change if you sail with Triton.
More than half a dozen companies share hands-on experiences this week in deep learning with the NVIDIA Triton Inference Server, open-source software that takes AI into production by simplifying how models run in any framework on any GPU or CPU for all forms of inference.
For instance, in a talk at GTC (free with registration) Fabian Bormann, an AI engineer at Volkswagen Group, conducts a virtual tour through the Computer Vision Model Zoo, a repository of solutions curated from the company’s internal teams and future partners.
The car maker integrates Triton into its Volkswagen Computer Vision Workbench so users can make contributions to the Model Zoo without needing to worry about whether they are based on ONNX, PyTorch or TensorFlow frameworks. Triton simplifies model management and deployment, and that’s key for VW’s work serving up AI models in new and interesting environments, Bormann says in a description of his talk (session E32736) at GTC.
Salesforce Sold on Triton Benchmarks
A leader in customer-relationship management software and services, Salesforce recently benchmarked Triton’s performance on some of the world’s largest AI models — the transformers used for natural-language processing.
“Triton not only has excellent serving performance, but also comes included with several critical functions like dynamic batching, model management and model prioritization. It is quick and easy to set up and works for many deep learning frameworks including TensorFlow and PyTorch,” said Nitish Shirish Keskar, a senior research manager at Salesforce who’s presenting his work at GTC (session S32713).
Keskar described in a recent blog his work validating that Triton can handle 500-600 queries per second (QPS) while processing 100 concurrent threads and staying under 200ms latency on the well-known BERT models used to understand speech and text. He tested Triton on the much larger CTRL and GPT2-XL models, finding that despite their billions of neural-network nodes, Triton still cranked out an amazing 32-35 QPS.
A Model Collaboration with Hugging Face
More than 5,000 organizations turn to Hugging Face for help summarizing, translating and analyzing text with its 7,000 AI models for natural-language processing. Jeff Boudier, its product director, will describe at GTC (session S32003) how his team drove 100x improvements in AI inference on its models, thanks to a flow that included Triton.
“We have a rich collaboration with NVIDIA, so our users can have the most optimized performance running models on a GPU,” said Boudier.
Hugging Face aims to combine Triton with TensorRT, NVIDIA’s software for optimizing AI models, to drive the time to process an inference with a BERT model down to less than a millisecond. “That would push the state of the art, opening up new use cases with benefits for a broad market,” he said.
Deployed at Scale for AI Inference
American Express uses Triton in an AI service that operates within a 2ms latency requirement to detect fraud in real time across $1 trillion in annual transactions.
As for throughput, Microsoft uses Triton on its Azure cloud service to power the AI behind GrammarLink, its online editor for Microsoft Word that’s expected to serve as many as half a trillion queries a year.
Less well known but well worth noting, LivePerson, based in New York, plans to run thousands of models on Triton in a cloud service that provides conversational AI capabilities to 18,000 customers including GM Financial, Home Depot and European cellular provider Orange.
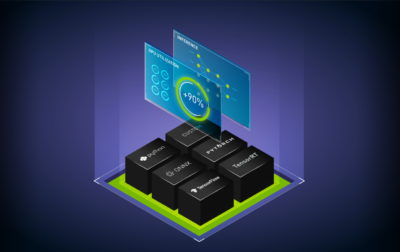
And the chief technology officer of London-based Intelligent Voice will describe at GTC (session S31452) its LexIQal system, which uses Triton for AI inference to detect fraud in insurance and financial services.
They are among many companies using NVIDIA for AI inference today. In the past year alone, users downloaded the Triton software more than 50,000 times.
Triton’s Swiss Army Spear
Triton is getting traction in part because it can handle any kind of AI inference job, whether it’s one that runs in real time, batch mode, as a streaming service or even if it involves a chain or ensemble of models. That flexibility eliminates the need for users to adopt and manage custom inference servers for each type of task.
In addition, Triton assures high system utilization, distributing work evenly across GPUs whether inference is running in a cloud service, in a local data center or at the edge of the network. And it’s open, extensible code lets users customize Triton to their specific needs.
NVIDIA keeps improving Triton, too. A recently added model analyzer combs through all the options to show users the optimal batch size or instances-per-GPU for their job. A new tool automates the job of translating and validating a model trained in Tensorflow or PyTorch into a TensorRT format; in future, it will support translating models to and from any neural-network format.
Meet Our Inference Partners
Triton’s attracted several partners who support the software in their cloud services, including Amazon, Google, Microsoft and Tencent. Others such as Allegro, Seldon and Red Hat support Triton in the software for enterprise data centers for workflows including MLOps, the extension to DevOps for AI.
At GTC (session S33118), Arm will describe how it adapted Triton as part of its neural-network software that runs inference directly on edge gateways. Two engineers from Dell EMC will show how to boost performance in video analytics 6x using Triton (session S31437), and NetApp will talk about its work integrating Triton with its solid-state storage arrays (session S32187).
To learn more, register for GTC and check out one of two introductory sessions (S31114, SE2690) with NVIDIA experts on Triton for deep learning inference.